Non-globular proteins in the era of Machine Learning
COST Action CA21160
research
Natural proteins encompass a broader spectrum of conformations, including structures such as: tandem repeat regions, modular architectures with a parallel folding pathway; intrinsically disordered proteins/regions (IDPs/IDRs), devoid of fixed 3D structure in their native state; aggregating domains, transmembrane proteins and proteins driving liquid-liquid phase-separation (LLPS). These proteins are usually known as “non-globular” proteins (NGPs) and they have shaken the long-held structure-function paradigm where well-defined native protein structures are needed for function.
NGPs participate in many biological processes of DNA and RNA binding, transcription, translation, cell-cycle regulation and signalling. NGPs also play a central role in numerous physiological and pathological processes, associated with misfolding and aggregation. They are implicated in a range of age-related diseases and systemic disorders such as Parkinson’s and Alzheimer’s, and type II diabetes. Recently it has also been discovered the important role of IDPs/IDRs in mediating LLPS and contributing to the formation of membraneless organelles.
The incredible advances in the field Machine Learning (ML) has revolutionized many fields of science, particularly life sciences, in the last years. One of the most widely known applications is AlphaFold, which was immediately recognized as a solution to the protein folding problem by the scientific community. However, there are still dark regions in the proteome involving NGPs that need to be addressed. The highly heterogeneous structural states and low sequence complexity of NGPs challenge not only the current experimental structure determination methods but also the state-of-art ML methods for structure prediction. A deep understanding of NGPs function requires the characterization of the conformational ensembles that they populate, which can be only achieved by integrating different biophysical experiments exploring various timescales with computational methods. Despite the demonstrated functional importance of NGPs, the available experimental data is still very limited compared to globular proteins, and/or lacks the standardization process to be properly stored. This fact hinders the generation of new large datasets which is a common need to then develop and train new ML approaches. This new era of highly accurate structure prediction and the application of ML approaches to solve biological problems claims for a timely action of the scientific community regarding NGPs, a field that needs the interplay between experiments and computation.
working groups
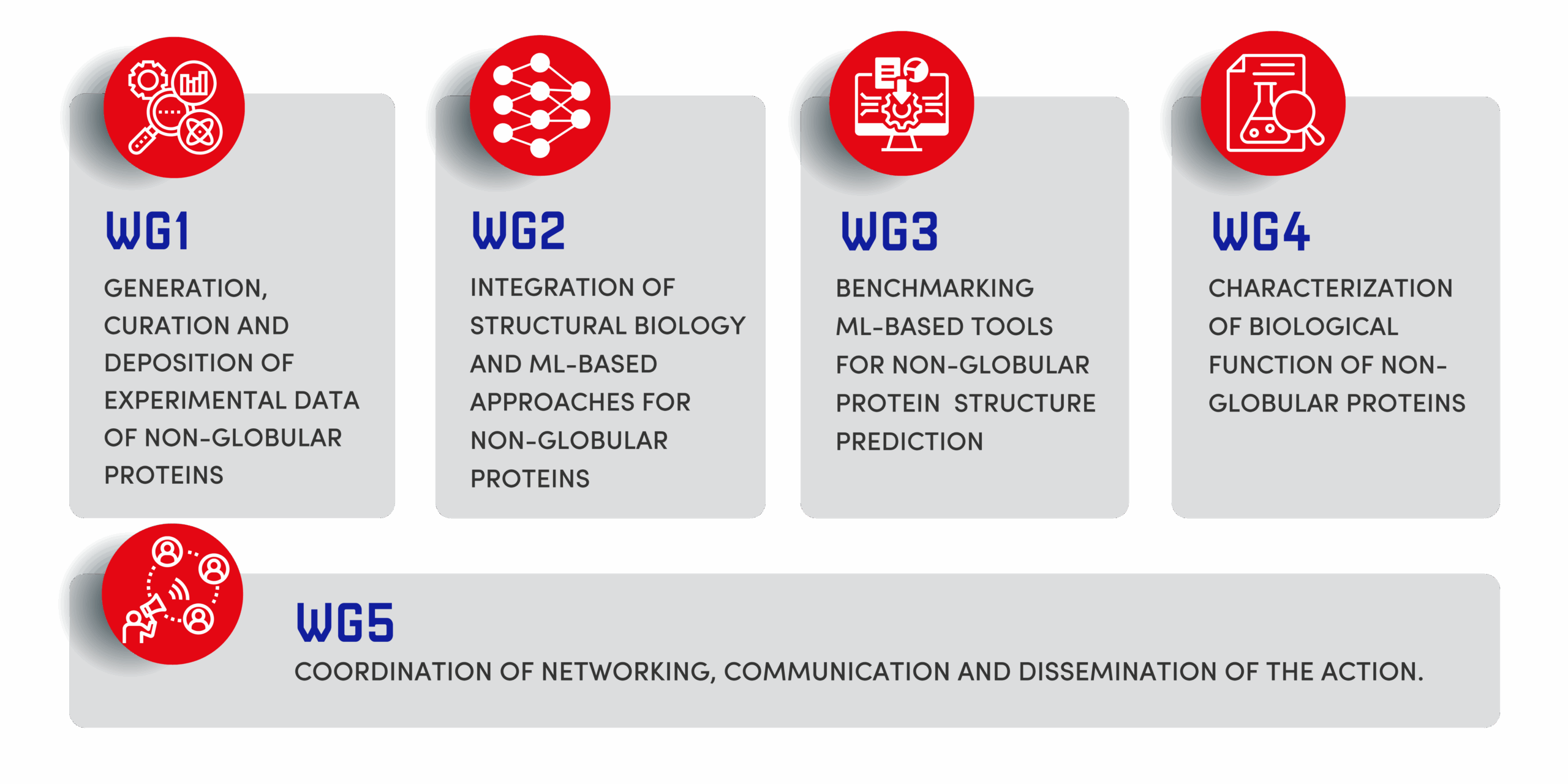
This WG is mainly focused on two key aims of this action: (i) Provide guidelines and best- practices to stimulate generation, curation and proper storing of NGP experimental data in order to significantly increase the available NGP experimental data; (ii) Generate open-accessible experimental datasets of different phenomena involving NGPs, with more quantitative, systematically collected and annotated data; (iii) increment the training-data for ML methods in the NGP field.
Leader | Dr. Pavel Kadeřávek
Co-leader | Dr. Dana Reichmann
The advance in ML approaches to protein biological function demands a cross-talk between computational methods developers and experimental methods. This WG will build-up specific approaches to integrate experimental methods used in the NGP field and machine learning approaches by promoting the interplay between these both sides, setting a place for networking and discussion and stimulating multidisciplinary activities.
Leader | Dr. Zsuzsanna Dosztányi
Co-leader | Dr. Javier Garcio-Pardo
This WG aims to build-up initiatives to critically assess state-of-the-art ML predictors and methods accuracy, developed in the context of the action but also by external contributors. This not only challenges the community to improve state-of-the-art methods but also contributes to the understanding of the strengths and weaknesses of each method. Synergies with actual critical assessments initiatives for globular proteins will be also sought in this WG.
Leader | Dr. Jovana Kovačević
Co-leader | Dr. Milana Grbic
This WG aims to take advantage of the data and methodologies produced by the other WGs to improve the biological function characterization of NGPs. The development of different pipelines and large-scale analysis will allow us to understand the major issues involving NGPs, such as: conformational heterogeneity, evolution, conserved motifs, protein-protein interactions, LLPS and involvement in neurodegenerative diseases.
Leader | Dr. R. Gonzalo Parra
Co-leader | Dr. Ana Melo
This WG aims to coordinate the networking, communication and dissemination of all WGs.
Leader | Dr. Rita VILAÇA
Co-Leader | Simone ATTANASIO